Artificial intelligence for multi-parameter optimisation of manufacturing procedures
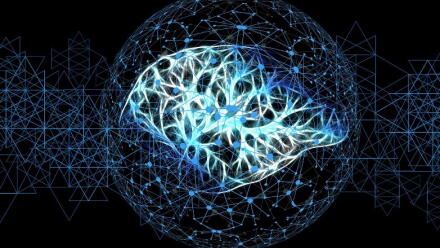
Impact Focus areas
Research area
Current global optimisation techniques involve three main approaches, (i) gradient based approaches, (ii) stochastic approaches and (iii) model-based approaches. When using a gradient-based approach, a large number of evaluations is required to determine which direction to go in. This is costly, time-consuming, and one can often get stuck in local minimum traps. Stochastic approaches scale poorly with each additional dimension/parameter. Model-based approaches require a large amount of prior knowledge, which may not be available for a particular problem, e.g. a new manufacturing process.
By leveraging novel machine learning techniques and heuristics, the novel approach from The Australian National University (ANU) enables efficient mapping/learning of the system to promptly generate candidate solutions, which optimises the system/process in question. This provides insights that are not normally available via existing methodologies/ approaches applied by human operators. The number of evaluations required is significantly reduced (machine learning requires less information to get to a solution), this approach scales very well with the addition of more dimensions/parameters and is able to avoid local minimum traps.
Potential benefits
- Efficient: Requires less information to get to a solution (including multiple parameter problems)
- Versatile: Can solve multi-parameter/high-dimension problems with one program
- Valuable decision making: Models generated can be utilised multiple times, to provide insight and deduce relevant system parameters
- User-friendly: Requires little to no specialisation or tuning
- Automated: Requires little to no human intervention/manpower
- Cheaper: Optimisation of your system/multiple processes will make your generation/manufacturing processes cheaper to produce
Potential applications
- Advanced/automated manufacturing
- Photonics (communications and manufacturing)
- High precision sensing
- Quantum technology (hardware through to sensing)
- Gravimetric analysis
- Hydrogen storage – optimisation of capture process
- Solar and wind – manufacturing of components
- Semiconductor – manufacturing of components
Opportunity
ANU is seeking industry and customer feedback for this technology (to ascertain which initial application(s) will suit this technology best), as well as engagement with industry partners/customers to work collaboratively with us to further develop the idea and optimise the algorithm for their specific application and/or manufacturing requirements.
Related publication: Multiparameter optimisation of a magneto‑optical trap using deep learning, Nature Communication, DOI https://doi.org/10.1038/s41467-018-06847-1
Key research team
Aaron Tranter, Department of Quantum Science, Research School of Physics
Contacts
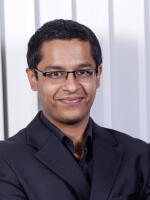